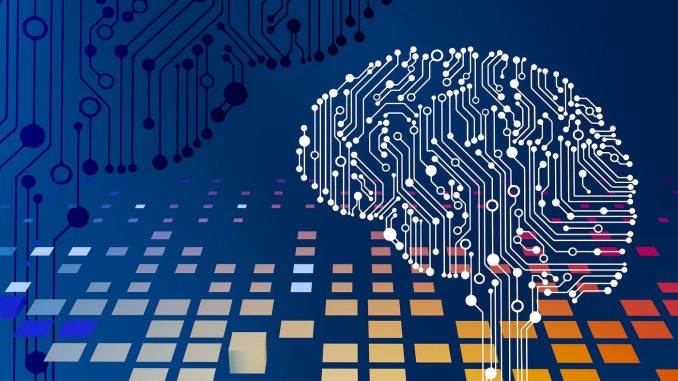
The innovative method of neuromorphic computing is built on technologies designed to mimic the operation of human brains for use in computer implementations.This creative method of neuromorphic computing has huge consequences for the future of AI.Besides, PMSPs will advance, adapt, and improve the efficiency.Pioneers in MPM and the Future of AI.In this article I will discuss neuromorphic computing and the future of AI.
Neuromorphic computing tries to translate the synaptic structures and functions of the human brain into computer hardware. Traditional computers are based on binary logic and sequential operation; neuromorphic systems, however, rely on spiking neural networks–asynchrony processing to some extent mimic living brains.This means that certain types of specialized hardware can radically speed up and optimize computational efficiency for neural operations.
Major advantages of Neuromorphic ComputingEnergy Efficient: One of the main attractions of neuromorphic computing is its energy efficiency. At present, deep learning-based AI systems have huge computational requirements and power demands. Contrast those with neuromorphic systems, which require only a small amount of power—Ideal for embedded and mobile applications where there is no extemal generator but many uses for such crosses in every direction.
Real-time Processing: Neuromorphic computing is good at real-time processing because it can handle asynchronous events and facilitates parallel processing. This feature is particularly useful in applications that need an instant response, such as self-driving automobiles, robotics, and real-time data analysis.Excellent Learning Abilities: In principle, neuromorphic systems are capable of enhancing the brain’s own learning change process via experience. These systems can take advantage of mechanisms such as spiketimingdependent plasticity (STDP), which allow them to learn from temporal data patterns; thus much more robust and adaptable AI models are enabled.
Scalability: The advantage of neuromorphic computing is that it can assist in the development of increasingly smart AIs. Neuromorphic hardware is modular, so it can take more processing units as it is required–a bigger or more efficient AI system does not by any means need extra resources for building abroad.
Applications and Impacts
Many applications exist for neuromorphic computing. Where is this technology expected to really gain a foothold? What are some of the first issues that will arise because of these points?
Autonomous Systems: In autonomous vehicles, the use of neuromorphic computing for sensor processing and decision-making will create safer and more reliable self-driving cars.
Robotics: Robots with neuromorphic processors can exhibit natural and adaptive behaviors more like humans, which helps them work out better among humans and do more complex tasks in changing environments.
Healthcare: In health care, there are several applications for neuromorphic computing. One of these is to perform accurate and efficient analyses of complex biological data so that personalized medicine can become widespread.
IoT Devices: When used in IoT devices, neuromorphic computing offers smarter and more efficient edge computing. By processing data in real time from the edge devices themselves, this means that sooner or later programmers will have to be back there where things are genuinely happening.
Challenges and Future Development
Despite its promise, there are a number of challenges that neuromorphic computing has to overcome. They include standard design methodologies, new programming paradigms and integrating these new systems in with existing computer infrastructures. Furthermore, more research needs to be put into whether or not neuromorphic hardware would offer long-term reliability and scalability before it starts to become common practice.
While the field continues to grow, physicists and engineers in it must transcend any difficulties they might presently face and to fully achieve the promise of neuromorphic computing. Now that hardware design, neural network algorithms and materials science have all made great strides, neuromorphic computing is expected to play a significant role in determining the future course of AI.
Neuromorphic computing, which mirrors the biological composition and information processing structure of our brains, is a completely novel route to creating AI. Because it can be done in real time, has higher energy efficiency and greater deep learning and scalability features, it is one kind of technology that can take on variety tasks. As research in this area progresses, it can afford the structure of manufacturing things in the AI systems of future generations. This may mean one entirely new dimension opens up for our sharpest future technologies, so that they take off yet again.