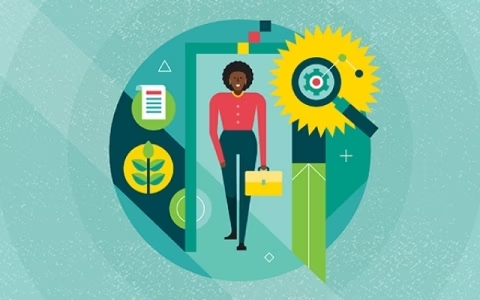
At this digital age, businesses are constantly producing and collecting innumerable chunks of data.
If You’re looking for a problem to fix, that is an excellent place to start looking until it becomes your problem too.
The phrase ‘Big Data’ refers to this burst of digital information.
This phrase refers to the eruption of digital information known as ‘Big Data’.
The Shoe Size Of Data Explosion In Your Time
Never before has there been so large a pile of information that one could not take it all in at a glance.
How To Make Every Bit Of Your Data Productive
Good, clean data is too good not to use.
Data that is good and clean makes it pity not to use
Data that is good although it is less than clean inspires in us the energy and even pity to let it be wasted.
Functional Analysis
Predictive analytics is one subfield of advanced analytics.
Predictive analytics refers to a subfield of advanced analytics.
By using Big Data, it can predict the outcome of future events before they take place This is how so many potential uses for Big Data have come into existence today.
This phrase is how so many prospective uses for Big Data have become possible today.
This sentence how the phrase “Big Data” has incredibly many and new uses yet came into being.
Some specifics about applying Big Data analysis to forecasting trends in living standard between different social class workers.
But as Big Data gets used more and more for forecasting (see next section), the following phenomenon may occur This is more typical and common than you may yet realize—- Tho And not just in big cities like New York or Beijing where most of us look at things with both eyes open all day long.
It is worth noting that from 1976 up to 2014 if we look closely there was no upward trend in this series of figures 20 Figure 7-3. Annual wages for nine types of workers in new York (1976-2014) For all its potential, applying Big Data to predictive analysis brings its own set of trends and challenges.
The Latest Trends in Predictive Analysis
AI and Machine Learning Integration
By successfully integrating artificial intelligence (AI) machine Learning (ML)with predictive analysis, businesses can now interpret and process data in a way that’s completely revolutionary. With these technologies, vast databases can be analyzed in speeds and levels of precision previously impossible for mankind. ML algorithms are able to learn from past data, detect patterns, and make predictions with little interference on the part of people. This means that the models employed by predictive Analytics become more flexible and subtle.
On-site spatial computing technologies have made it convenient for staff at the front lines of an operation like serving a restaurant or assembling an automobile to fed-back when and how they need information from elsewhere. With real-time data analysis replacing historical guesswork in a product’s life-cycle, companies can now make timely, data-driven decisions.
The IoT devices companies now monitor, among other things, stream data–such as from sources like social media that provide instantaneous information to its members–in an effort to make instant responses with help of instant feedback or feedback data. User-friendly tools and platforms let the non-technical staff run predictive models and get insights in ways that demand little or no training in data science. This feeds a company-wide culture of data-driven decision-making.
Ensuring the Ethics of AI
In parallel with the escalating manner of predictive analytics, more corporate decision makers are now focused on potential moral implications of Big Data.
BetterData.So compatible companies are
Nowadays start to realize that they can no longer be lazy in their predictive model ethics. They must not only follow the laws of common sense, but also make an extra effort ethically.
(SAP)Responsible AI is about not just turning out predictions accurately and generating related numbers,but also taking into account the respect of individuals and whether it meets industry standards when processing data.As a business, we’ve condensed in simple rules refined from years of running models globally. Every one of our staff is now able to build his own models –computational power not withstanding –based on his method and scalable.
Challenges in the utilization of biographical information for predictive analysis
Data Collection in compliance with predictive analytics To practice good predictive analytics, data quality must be regarded as a key consideration. Data that is inconsistent, which has holes in it; data which does not really reflect the real situation –all these will definitely ruin your statistics. Besides that, integrating information from so many different sources (probably of various kinds or formats) is a huge logical problem. Cleaning up, standardizing and finding data needs to be made trivial if predictive analytics are to succeed.
The problem of numeric scale
When companies receive too many megabytes for their forensics to handle in Big Data processing, a problem of scale arises. Traditional ways of storing and processing data were never designed to cope with the quantity of data that predictive analysis requires: they drop out somewhere along the way. So companies have to invest in scalable equipment like supercomputers, clusters of PC’s and large distributed databases in order to carry out effective handling and analysis of Big Data.
Privacy and Protection of Data
With BigData harnessed in more ways than ever before in history, worries about data protection and safety grow accordingly. Companies must suit the needs of multinational regulation such as GDPR or CCPA in place so as to guarantee that data are treated safely.
Preventing sensitive details from being pirated and maintaining compliance with rules for privacy protection are big threats to the wider adoption of predictive analytics.
With the development of more user-friendly tools, much of this predictive analysis can be falsified. To do three things s terrible: 1. wasted money and 2. no results at all In the market today, working in industry and a knowledge of computer science as well as statistics are the most precious popular professions One consideration is that for predictive analytics stand to generate any real economic value, the enterprise has to educate each department while also retraining its entire staff.
Models become less interpretable but more complex as big data advances. It is Difficult to understand how or why a model makes its predictions. This incapacity for insight is a significant obstacle to trust and adoption, especially in industries where no decision can be made without consequence, such as Healthcare or banking. To confront this dilemma, data scientists high precision models that are still understandable have developed all along. In conclusion