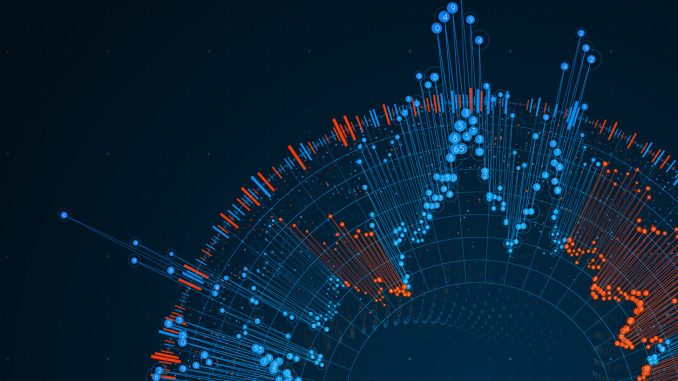
If You’re looking for a problem to fix, that is an excellent place to start looking until it becomes your problem too. The phrase ‘Big Data’ refers to this burst of digital information. The Shoe Size Of Data Explosion In Your Time It has never been so large a pile of information that it could not be viewed whole .How To Make Every Bit Of Your Data Productive Data that is good and clean makes it pity not to use. Functional Analysis Predictive analytics refers to a subfield of advanced analytics. In advance of events happening, it uses Big Data to forecast likely outcomes This phrase is how so many prospective uses for Big Data have become possible today Some specifics about applying Big Data analysis to forecasting trends in living standards among different social classes of workers For all its potential, applying Big Data to predictive analysis brings its own set of trends and challenges.
The Latest Trends in Predictive Analysis
AI and Machine Learning Integration The successful combination of artificial intelligence (AI) machine learning (ML) with predictive analytics is changing the way that businesses interpret and process data. These technologies make it possible to analyze enormous databases at rates and levels of accuracy previously unattainable. ML algorithms can learn from past data, find patterns and forecast with minimal human interference. This means that the models put to work by predictive analytics become more dynamic and adaptive.
Real-Time Analytics As businesses ratchet up the pursuit of agility, the demand for real-time analysis is intensifying. Companies are now picking up streaming data from IoT devices, social media and other sources of instant information to make instant, data-based decisions. With real-time predictive analytics, companies can rapidly adjust product lines and final assembly systems and give customers tailored experiences.
Data Democratization More and more employees in companies are following the trend toward data democratization. User-friendly tools and platforms allow non-technical staff members to run predictive models and draw insights in ways that require little know-how of data science. This trend is fostering a company-wide culture of data-driven decision-making.
Ensuring AI’s Ethical nature
Complementing the ever-growing sophistication of predictive analytics, more and more corporate executives are paying attention to the ethical implications of Big Data. Organizations now recognize that they cannot afford to take the shortcut to ethics in prediction models but they must stick to the rules of common sense and also work harder ethically Responsible AI means not only producing accurate forecasts and related results, but also respects the privacy of people as well as meets industry standards for the use of data.
As an enterprise, we have summed up our lessons from successful deployment of international models so that without having privacy problems or other negative aspects to watch out for—all staff may build their own models based on their methods scalable and easily.
Challenges of Utilizing Biographical Data for Predictive Analysis
Data Collection in compliance with To engage in good predictive analytics, data quality must be considered key. Data that is inconsistent or has holes in it; data which is not an accurate representation of reality will definitely lead to statistics. Furthermore, integrating information from many different sources (some very possibly of different kinds or formats) is a technical challenge of great logic. Cleaning, standardizing and finding data must be made elementary for successful predictive analytics.
Numerical scale problem
When companies receive so many megabytes of Big Data processing for their forensics to handle, they have a scalability issue. Traditional methods of storing and processing data aren’t made to cope with the large data volume needed for predictive analysis: they break down somewhere along the line. Accordingly, companies have to invest in scalable infrastructure such as cloud computing as well as large-scale distributed databases in order both to manage and analyze Big Data effectively.
Data Privacy and Protection
With Big Data being used in more ways than ever before in its history, concerns regarding data privacy and protection grow proportionally. Organizations must navigate the complicated waters of multinational regulatory requ irements such as GDPR and CCPA so as to ensure that people’s personal information is handled safely. Preventing sensitive information from being hacked and keeping pace with the rules for protecting privacy both pose major threats to widespread adoption of predictive analytics.
Despite user-friendly tool advances there is still a great deal of predictive analysis lies ahead; meanwhile inaction means wasted money and no results at all. Data scientists, who have knowledge in computer science and statistics, are in great demand and short supply. It is necessary for enterprises to educate its departments and retrain the entire staff if the full potential of predictive analytics is to be exploited.
In conclusion
Model Interpretability Models become more complex as predictions advance. Making sense of how and why a model puts out its predictions is difficult. Such incapacity for understanding is a major obstacle to trust and adoption, especially in industries where no decision can come without consequences — for instance, healthcare or banking. All along–this is the direction in which data scientists have been working, is to develop models that are accurate but also interpretable.